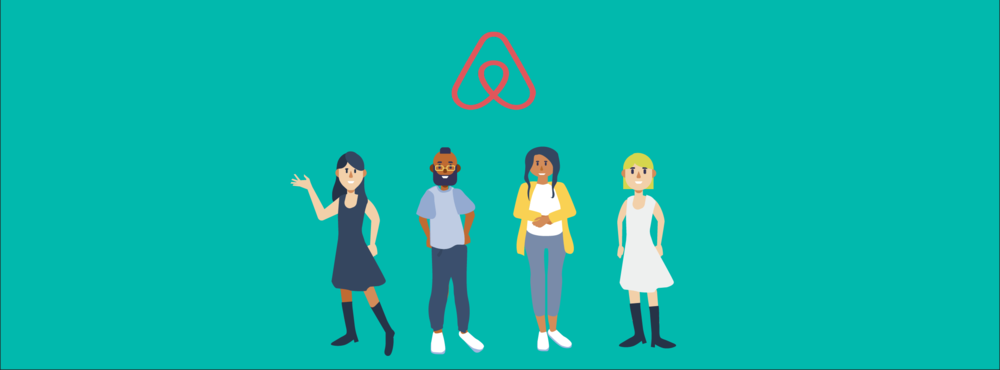
About the Data
Data provided on the online platform Inside Airbnb were sourced from publicly available information from the original Airbnb site, according to background information on the Inside Airbnb website. The data were cleansed and formatted by the creators of the site into a user-friendly, visually appealing form. The website contains datasets pertaining to Airbnb listings in relatively populated and touristy places across the globe such as Beijing, Berlin, Copenhagen, and Los Angeles. For the purpose of our project, we will be focusing on the dataset of Los Angeles Airbnb listings.
The creator of the dataset chose to compile information including metadata such as ID and Host ID, categorical data such as the name of the listing and neighborhood, as well as quantitative data such as room type and price, minimum nights per stay, number of reviews per listing per month, availability of listings, and how many listings the host maintains. Data was generated and handled using Python, jQuery, Leaflet, and Mapbox Studio.
Sources and Funding
Inside Airbnb allows people to see how Airbnbs are utilized around the world by analyzing the data in accessible tables and visually aesthetic graphs/maps. The original data sources are from Airbnb’s public listings, which are gathered from the Airbnb website. Inside Airbnb’s “About” page mentions that the data site is not affiliated with Airbnb. As indicated in the “About” page, there may be “spam” reviews; however these reviews have a negligible impact on the overall statistics. This data comes from a snapshot of past listings and depicts these past rental locations worldwide.
Murray Cox is the founder, creator, and a primary funder of the Inside Airbnb project. Cox is a storyteller, community activist, and technologist, who utilized these aspects of his identity to compile and analyze the data, as well as build the Inside Airbnb website. Cox received help from John Morris, a graphic designer, for the creation of the data’s design and user experience. Cox’s goal in compiling the data is to facilitate public discussion of this data, as his intended audience for this dataset is the general public. Inside Airbnb is funded primarily by Cox, along with donations that users can make on the website. Donations fund the collection of data, costs of technology, running the website, as well as compensating project workers.
Processing
Before we could begin exploring our dataset and constructing our narrative, it was necessary to clean the initial dataset since it was far too large to be used effectively in data analysis and visualization, and contained some variables that were extraneous or lacking meaning. In order to reduce the dataset to a usable, manageable size, we removed variables that we considered irrelevant to our early research interests, including metadata like the link to the listing and the date that the listing page was last scraped. Although we could have further consolidated the dataset as well as narrowed the scope of our project by considering only Los Angeles listings and excluding listings from other cities and unincorporated areas, we instead chose to examine data from all 3 of these neighborhood groups. This is because we felt that having access to all 3 neighborhood groups of Los Angeles county would aid us in exploring topics of gentrification, and the varied impact AirBnb may have based on varying socioeconomic status. We also chose to remove columns containing dense textual data from the dataset, such as name and description of listing, and the host’s about me section, with the intent to examine this textual data in isolation from the rest of the dataset, using Voyant Tools and other textual analysis. Furthermore, as we cleaned and prepped our data, we utilized Breve and Data Refine in order to identify gaps, silences, and inconsistencies in the data; we discovered that License, a categorical column that contains license numbers, exemptions, and blanks, consisted primarily of blanks or nulls in the data. We furthered explored this data silence in our Insights and corresponding narrative.
Presentation
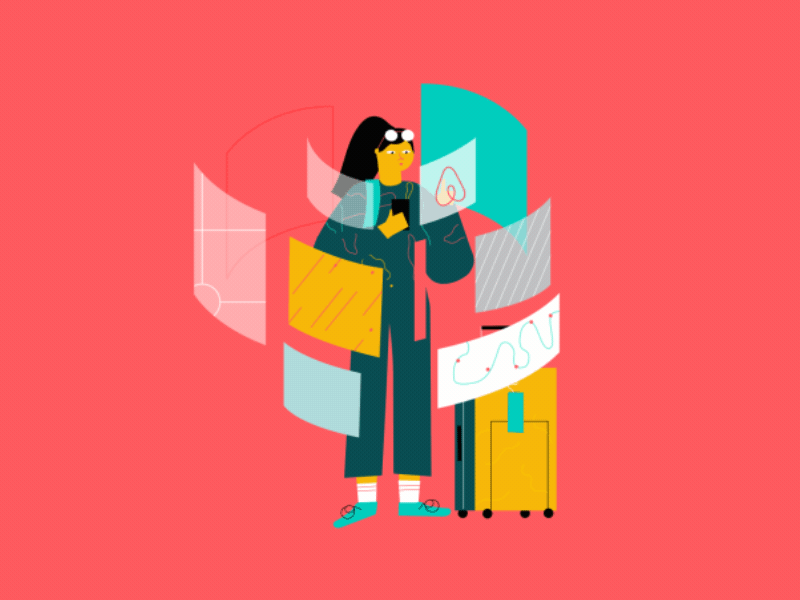
In this project, our main purpose is to provide a humanistic perspective of this business-oriented data, especially on the biases in the service itself, and how it effects on the long-term rental market, and gentrification. We serve as narrators in this phenomenon to analyze how theses effects are shaping the socioeconomic well-being of the city and the history of humanity.
For the designing aspects of the project, our team decided to present the information in a similar way that users will navigate on the Airbnb site. We particularly utilized color palettes, typography etc that are identical to the Airbnb site. Our team also ensured that the visual presentation is simple, so users can effortlessly comprehend the information on the website.
In regard to our data visualizations, most of them are created on Tableau. Not only are Tableau visualizations interactive, they are also user-friendly and demonstrates the correlations of variables very clearly. Data visualizations are important because it allows us to make comparisons and identify patterns in the data. In addition, our team also used Voyant tools for our visualizations, which is a web-based reading and analysis environment for digital texts. We used the application to identify the textual data of Airbnb reviews in Los Angeles. This is particularly useful as it provides a higher understanding of the review biases in the platform.
Additionally, we used WordPress to build and design the website. WordPress is an easily accessible web design application that is free, user-friendly and has a variety of templates and plugins available.
WEB TOOLS OVERVIEW
- The website was constructed through WordPress.
- The data visualizations were generated by using Tableau, and Voyant tools.
- The timeline is created using Northwestern University Knight Lab’s Timeline JS framework.
Data Critique
Information Provided by the Dataset
Inside Airbnb’s datasets are stored in .csv files regarding listings, calendar information, customer reviews, and summaries of listing metrics across different regions and cities across the world. The dataset also includes .geojson files to give information about the neighborhoods of the city in focus.
Delving deeper into the specifics of the data, the data include the name and description of the Airbnb, the neighborhood where it is located in, the room types, amenities, price per night, occupancy rate, and also pictures of the Airbnb. The dataset also included information about the hosts, their response rate, occupations, and where they are located. Furthermore, the dataset can illustrate the standard of evaluating a profitable Airbnb by considering different metrics, such as the occupancy rate, total number of reviews and rating score of the reviews. It also illustrates how metadata can be gathered to cater to people’s different needs. By selecting requirements as detailed as to how many bathrooms there are or whether the house will allow pets, customers with varying needs can utilize the metadata provided by different hosts to their convenience to accommodate their ideals for their stay.
By honing in on the dataset for Los Angeles, we can observe one of many data maps consisting of points which disclose different room types (specifically whether the listing is a home or apartment, private room, or shared room). The data can be visualized using multiple filters, as zooming in further on the map to a single point shows specific information, such as an Airbnb’s availability for rent. Different points on the map also include reviews from previous clients.
The goal of the data is to illustrate the effects Airbnb may have as a business. In particular, our team wants to illuminate the harmful effects that Airbnb may have on multiple issues: gentrification, housing market (reasonable, affordable housing prices), illegal listings, minority communities. We believe that Airbnb could potentially be hurting the economy, using our data to disagree with Airbnb’s statement of helping the “sharing economy.”
Limitations of the Data
In terms of the limitations of Inside Airbnb’s dataset, their dataset does not reveal private information. Some data is missing in terms of personal information about the guests and hosts including: ethnic background, gender, levels of income, age of individuals, profession, and education levels. Some qualitative data of the location itself is missing, including weather, earthquake or hurricane risks, neighborhood safety, and crime indexes. There is also no data in politically charged areas, such as South Korea, Iran, and Syria. Also, some locations have stricter laws than others as to who can or cannot rent and/or host.
Moreover, both booked and unavailable spots are clustered together as ‘unavailable nights’. Even though the data does not directly depict gaps between minority communities, the silence of ethnic backgrounds may show the inequality in wealth. The data seems to give little information as to the identification of the individual or group of renters, yet this is clearly for privacy reasons. However, there is generally more information given about the host.
The spreadsheet also does not include exact location information, as Airbnb listings’ location information are anonymized, meaning that the location for a spot on a map or in the data will be 0 to 450 feet from the actual address. Additionally, the data provided by Inside Airbnb are a snapshot of the Airbnb listings at a specific time. Hence, some of the Airbnb listings included in the dataset may have been deleted, or some Airbnb listings may have been added since the snapshots were made.